2022
Index:
Natural structures inspire the design of new lightweight metamaterials
Machine learning boosts shape memory polymer materials research
Learning Experiences Boosting STEM Education and Interest in STEM Careers
High Entropy Alloy as Enhanced Hydrogen Catalyst
Discovery of Cellular Unit Cells with High Natural Frequency and Energy Absorption Capabilities by an Inverse Machine Learning Framework
LAMDA+: Leveraging research projects by engaging on-campus intellectual properties
Curing Behavior Simulator for Robotic 3D Printing of UV-Curable Thermoset Polymers
A soft syntactic foam actuator with high recovery stress, actuation strain, and energy output
A thermoset shape memory polymer based syntactic foam with flame retardancy and 3D printability
Success with Research Experiences for Undergraduate Students During Pandemic Restrictions
An Atomistic-informed Continuum Model for Materials Microstructural Design
Room-Temperature Self-Healable and Mechanically Robust Thermoset Polymer for Healing Delamination and Recycling Carbon Fiber
From drug molecules to thermoset shape memory polymers: A machine learning approach
Real-time sensors developed to improve quality of 3D printed parts
Synergistic Research, Education, and Outreach through Undergraduate Projects in Additive Manufacturing
2021
Cutting-edge technologies for interdisciplinary materials research
Accelerating deep learning discovery of new thermoset shape memory polymers
Phosphate esters dynamic chemistry enables flame retardant vitrimers
Recyclable Composites: The future of lightweight materials
Inverse machine learning technique optimizes discovery of materials for sandwich structures
New specimen design improves rapid fatigue testing
Developing artificial intelligence for defect detection
Virtual Training Modules for Additive Manufacturing Bridges Knowledge Gaps During COVID Closures
2020 and earlier
Natural structures inspire the design of new lightweight metamaterials
by Amber King, Louisiana EPSCoR (View the newsletter as a PDF)
You wouldn’t expect to hear about hedgehogs and porcupines in a materials science research lab, but their spines and quills are very much of interest because they have superior resistance to buckling, while also being hollow and lightweight.
Nature has perfected structure specialization in so many ways and researchers have realized that investigating new materials benefits greatly with “biomimetics,” which is the field of science that draws from biological processes and designs found in nature.
The menu of nature’s finest engineering designs is extensive and the combinations of strengths and benefits are unlimited. For example, combine the buckling resistance of hedgehog spines, the strength-to-weight ratio of bamboo— which is very light and can withstand up to 52,000 pounds of pressure per square inch— and the tough scales of fish that provide vibration damping and streamlined hydrodynamics, and you could possibly design significantly stronger, lighter, faster, and more fuel-efficient aircraft fuselages, ship hulls, and car bodies.
Louisiana Materials Design Alliance (LAMDA) researchers are using biomimetics in the design of new structures that solve engineering problems relating to strength, weight, durability, and more. LAMDA is funded by a $20 million cooperative agreement with the National Science Foundation and support from the Louisiana Board of Regents.
Specifically, LAMDA researchers are designing “sandwich structures,” that are thin-walled cellular structures and lattice designs sandwiched between two thin outer skins. These composite materials are lightweight and strong due to the structure of the core of the sandwich, which can vary anywhere from a simple grid to complicated 3D shapes. The goal is to design new cores that can be used to manufacture the lightest and strongest sandwich structures.
Photo: Mr. Adithya Challapalli, LSU Ph.D. student, is holding several optimized lattice cores 3D printed using a TSMP ink.
But it doesn’t stop there. The team is researching lightweight structures with an extra feature of being made out of 3D printed Thermoset Shape Memory Polymers (TSMP). These highly specialized polymers are “smart” materials and have “shape memory,” the capability of returning to its original shape after being deformed or triggered by certain stimuli. This added smart property categorizes this class of new materials as “metamaterials.”
The challenge is that taking inspiration from nature and testing each design with classic numerical analysis techniques is a very time consuming endeavor due to the millions of possible structural combinations.
LAMDA Research Assistant and PhD student at LSU, Adithya Challapalli, is taking the TSMP design to the next level with machine learning. He developed a generative adversarial network (GAN) and regression analysis to discover the correlation between the microstructures and mechanical properties of biomimetic rods and then further optimized the biomimetic rods into lattice and cellular structures as a sandwich core. In addition, he developed a new inverse machine learning framework to speed up the process of discovering new metamaterials with superior properties.
“Mr. Adithya Challapalli developed a new inverse machine learning framework, and successfully discovered several new metamaterials with mechanical strength, natural frequency, and impact tolerance superior to their natural counterparts, such as honeycomb,” said Dr. Guoqiang Li. “Most interestingly, he discovered several new lattice structures and thin-walled cellular structures, 3D printed them using TSMP, and proved that these structures have record-high recovery stress. His inverse machine learning framework has a good potential to optimize other engineering structures and devices,” added Li.
Challapalli is in the last semester of his doctorate program, and is appreciative to Dr. Li, Major Morris S. & DeEtte A. Anderson Memorial LSU Alumni Professor and John W. Rhea, Jr. Professor of Mechanical Engineering at LSU, and Distinguished Research Professor at Southern University, for providing guidance and the freedom to explore different research ideas in this multidisciplinary field, and bringing together collaborators in material science, structural mechanics, and computer science.
“I believe the inverse design frameworks and machine learning techniques proposed through my research can be extended and be used as an alternative data-driven optimization technique. The lightweight structures discovered through these frameworks are much superior to previously designed structures in terms of load-carrying capacities, buckling and impact strengths, energy absorption and shape recovery properties with applications in aerospace, automobiles, naval vessels etc.,” said Challapalli.
Challapalli’s imminent completion of his PhD in Engineering Science with a Materials Science & Engineering concentration will be a great complement to his B.S. in Mechanical Engineering from India and M.S. in Mechanical & Energy Engineering from University of North Texas. He is currently looking for a job in the industrial sector as a mechanical designer or research and development engineer.
Figure: Step-by-step optimization by machine learning for discovering cores for lightweight sandwich structures.
Machine learning boosts shape memory polymer materials research
by Amber King, Louisiana EPSCoR (View the newsletter as a PDF)
Imagine you are packing for a long trip overseas, and you find your suitcase is entirely too small, your items are too heavy for the weight restriction, and you still have very expensive and fragile mechanical items yet to pack. Expand this problem to the size of a rocket, and you have the biggest engineering conundrum for space travel.
Scientists have developed an ingenious solution: Shape memory polymers. Let’s say a solar array for a satellite is being packed into a rocket. Shape memory polymers can replace the delicate hinges and mechanical gears required to open the panels. This allows the system to be packed highly compressed, weigh significantly less, and with no fragile metal parts. After the rocket reaches orbit, the compacted array can be launched, and when it is warmed by sunlight, voilà!, the shape memory polymers are activated and gently open it without a single gear. This technology was successfully employed with the SJ20 Geostationary Satellite launch in 2019.
Shape memory polymers (SMPs) are special synthesized materials that change shape when “activated” by a trigger such as heat, light, electricity, enzymes, magnetism, or pH solution. This material can be used in self-healing materials, parts of a soft robot, as an artificial muscle, geothermal drilling proppant, etc.
Dr. Cheng Yan has been applying his expertise in establishing SMP physical models by solid mechanics and leveraging machine learning to discover new SMP materials at Louisiana State University (LSU). He is a postdoctoral researcher as part of a collaborative research team with LSU and Louisiana Tech University researchers for the Louisiana Materials Design Alliance (LAMDA).
Photo: LAMDA postdoctoral researcher Dr. Cheng Yan works on a paper on one computer and runs machine learning codes on the laptop at Louisiana State University.
The LAMDA research alliance is funded by a $20 million cooperative agreement with the National Science Foundation and support from the Louisiana Board of Regents.
Machine learning is a sub-field of artificial intelligence, which is a powerful tool to endow the computer to learn and make decisions with less human involvement. Machine learning is able to overcome the brute force approach in traditional material discovery, thus saves a lot of time and money by allowing researchers to experiment virtually first, and then physically synthesize desired new materials in the lab.
But first, the appropriate machine learning algorithm has to be chosen, which requires the deep understanding and expertise for both material science and computer science. This being a relatively new field, there are not readily available databases for TSMPs yet, and manually collecting datapoints is quite time-consuming.
To solve this problem, a variational autoencoder model was trained to borrow knowledge from already-researched drug molecules from a big drug database and a small TSMP monomer/crosslinker database to find the desired new UV-curable TSMPs.
“Dr. Cheng Yan was the first to use the concept of transfer learning in solving the challenge of small training dataset in TSMPs. He successfully predicted five new types of TSMPs, and one of them has been validated through synthesizing and testing,” said Dr. Guoqiang Li.
“His machine learning framework not only works for discovering new TSMPs, but also works for guiding the design of other new polymers. These polymers have a great potential to be used in various engineering structures and devices,” added Li.
Illustration: (Left) A polymer must have a stable network and a switching phase to possess shape memory effect. (Right) A schematic showing the thermomechanical cycle. Source: H. Meng and G. Li. Polymer 2013, 54, 2199.
Yan’s research has contributed to 11 published papers in leading scientific journals in five years, and as a lead author or corresponding author for 10 of the papers, which is quite an accomplishment. He also was awarded “Best Paper” at the 16th International Conference on Computer Science and Education.
The LSU Mechanical & Industrial Engineering Department recently recognized Dr. Yan for these achievements with the 2021-2022 “Outstanding Research Assistant” award.
Math has been at the core of Yan’s interests since he was a child in Xinjiang, China. “I have always been interested in math. Math is the foundation of engineering, and we use math to translate physical problems in engineering. Just like Galileo Galilei said, ‘Mathematics is the alphabet with which God has written the universe,’ ” explained Yan.
Dr. Yan credits his mentor and advisor, Dr. Guoqiang Li, LSU’s Major Morris S. & DeEtte A. Anderson Memorial LSU Alumni Professor and John W. Rhea, Jr. Professor of Mechanical Engineering, for his motivation to research SMPs in Louisiana. “I read two papers about SMPs by Dr. Li in the Journal of the Mechanics and Physics of Solids, which I consider the best journal in solid mechanics, and decided right then that I wanted to be part of this research effort,” said Yan. “Dr. Li has been a good example for me and has given me a lot of motivation to go forward. We can learn a lot from him,” he added.
The next phase of Dr. Yan’s career in Louisiana continues, as he is preparing to start a new position as an Assistant Professor at Southern University and A&M College’s Mechanical Engineering Department.
Learning Experiences Boosting STEM Education and Interest in STEM Careers
by Amber King, (View newsletter as a PDF)
The Louisiana Materials Design Alliance (LAMDA) recently launched a seed funding program for K-12 STEM outreach projects relevant to the Louisiana Student Standards for Science, the LaSTEM statewide goals and objectives, and/or LAMDA’s research themes of machine learning, experimental and computational materials science & engineering, and additive manufacturing. LAMDA is an NSF EPSCoR RII Track-1 project striving to propel the State of Louisiana to a leading and sustainable position in advanced manufacturing and materials development. The first projects selected for seed funding reach across the state and are as follows:
Exploring Novel Manufacturing Technologies for Lightweight Material Design in Mechanical Engineering
Louisiana State University (LSU) is partnering with the Denham Springs High School STEM and Robotics Center to provide mechanical engineering workshops to high school students. Dr. Geneveive Palardy, Assistant Professor of Mechanical Engineering, and a team of graduate and undergraduate students, will develop and present this new workshop series designed to raise awareness of STEM education and career opportunities in the field of composite materials.
The first workshop will introduce students to photopolymers and polymer composites, as well as novel 3D printing technologies and the basic steps for design. Students will be assigned a mini-Capstone team project to design a lightweight, yet sturdy, vehicle out of the different materials and printing technologies available to them. Additional sessions will be delivered to allow the teams to make progress on their design projects, ask questions, and receive assistance about concept ideas, CAD modeling, 3D printing, and testing. At the conclusion of the series, the teams will have a friendly competition for the best and most lightweight design.
After the workshop, some of the printers will be donated to the partner school to allow them to continue implementing similar activities for future students.
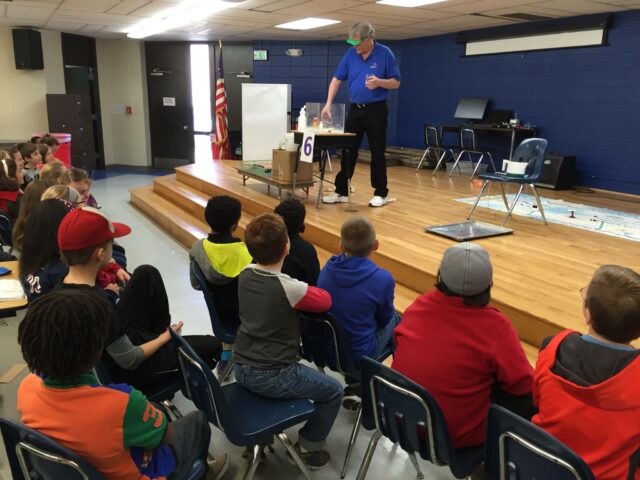
Making a CASE (Collaborating Around STEM Engagement) for Middle Grades
Louisiana Tech University (LATech) will provide multi-disciplinary outreach to middle grade students in Lincoln Parish to stimulate interest and improve basic knowledge in physical science. The CASE program is led by co-PIs Dr. William Deese, Professor of Chemistry, and Ms. Cathi Cox-Boniol, LATech STEM Center Director.
“We are excited to have a LAMDA STEM Outreach award! It provides us with the opportunity to conduct on-campus activities this spring. Our goal is to stimulate interest in science and STEM careers with middle grade students in Lincoln Parish. Understanding how science is done (the scientific method) is extremely important in this age group. We believe we have put together a great program that will achieve these goals,” said Deese.
The key experiences will be presented during STEM days on the LATech campus, where the students will tour virtual and physical state-of-the-art facilities. Students will be part of several interactive workshops on the scientific method and matter and its interactions. Students will also meet LATech STEM professionals from diverse backgrounds and hear their unique journeys and lessons learned in their STEM profession. In addition, teachers will receive mentoring and materials to continue the experiences in the classroom.
Additive Manufacturing Summer Camps
Southeastern Louisiana University (SELU) is leading two-week-long summer camps for underserved, underrepresented minority students from Pine High School and St. Helena College & Career Academy. The PI, Dr. Mehmet Bahadir, Assistant Professor of Industrial Technology, has developed partnerships with Northshore Technical Community College (NTCC), and Northshore Regional STEM Center for these summer camps.
During the first week of the camp, the students and teachers will be trained on 3D printing technologies, 3D computer modeling, and the engineering design process with the Makerbot Print Certification program, earning participants an Operator Certification and an Innovator Certification. In the second week, students will apply their new skills toward a 3D modeling and printing challenge. The challenge experience will culminate with a field trip to NTCC Lacombe STEM campus and SELU’s new Computer Science & Technology building.
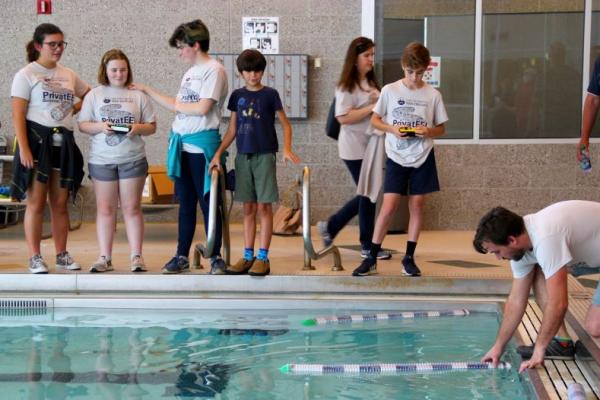
Robotic Eel Summer Camp
The University of New Orleans (UNO) is expanding their successful Eel camp to the teachers and students at St. Charles Borromeo School in Destrehan.
“This camp exposes middle school kids to various concepts in engineering including: 3D CAD design, 3D printing, hydrodynamics, electrical design and mechanical design using a fun, hands-on approach,” said the PI, Dr. Brandon Taravella, Professor in the School of Naval Architecture and Marine Engineering.
During the five day camp, students will be given lessons in using Solidworks 3D modeling and printing software. Campers will get a tour of the UNO campus and engineering facilities. After introductory lessons in hydrodynamics, the students will perform tests in the Towing Tank.
The campers will then be given 3D models of the parts for the eel robot. They will be able to modify the design to improve the hydrodynamic performance and then print the parts for assembly. After programming the eel robots, they test them in the Towing Tank and prepare for the final robot sprint and obstacle course competitions in a swimming pool.
High Entropy Alloy as Enhanced Hydrogen Catalyst
F. McKay, Y. Fang, O. Kizilkaya, P. Singh, D. Johnson, A. Roy, D. Young, P.T. Sprunger, F. Flake, John; S. Shelton, Y. Xu, Louisiana State University
High-entropy alloys (HEAs), equal concentration multimetals, have intriguing material properties, but their potential as catalysts has not been widely explored. Louisiana State University (LSU) and NASA’s Ames Research Center researchers show how a high entropy alloy of Cr, Fe, Co, and Ni significantly improves hydrogen evolution activity vs. the individual base metals.
Photo: Louisiana State University (LSU) Physics graduate student Frank McKay conducting LAMDA catalyst research at the LSU Center for Advanced Microstructures and Devices (CAMD).
Impact
Hydrogen evolution reaction (HER) is key to renewable energy production. Pt is the most active element that catalyzes HER, with high expense and limited availability. This work shows that multimetallic alloys offer a large design space where compositions based on common elements may exist that mimic critical metals for specific catalytic reactions.
Background
Catalysis is at the heart of energy conversion and chemical manufacturing technologies. Environmental sustainability demands innovative, affordable, and active catalytic processes. While the platinum group of metals have always played a significant role in catalysis, the need to replace them with Earth-abundant elements is keenly recognized by the U.S. government. High entropy alloys (HEAs) with designed compositions can be nanoengineered to mimic these precious metals and create multifunctional catalytic surfaces.
We demonstrate that a quaternary HEA composed solely of the 3d base metals Co, Cr, Fe, and Ni exhibits activity for the electrochemical hydrogen evolution reaction (HER) that approaches the activity of Pt. Crystalline CoCrFeNi random alloy is tested for the HEA for electrochemical HER used as a probe reaction for its simplicity and technological utility. Based on both electrochemical and theoretical studies, the results demonstrate that pure Ni sites are more resistant to oxidation than sites composed of the other metals, and that a partially oxidized HEA surface is the active state of the surface for catalyzing the HER.
Discovery of Cellular Unit Cells with High Natural Frequency and Energy Absorption Capabilities by an Inverse Machine Learning Framework
Adithya Challapalli and Guoqiang Li, Louisiana State University
Machine learning regression and Generative Adversarial Networks (GANs) were used to form an inverse design framework. Optimal cellular unit cells that surpass the performance of biomimetic structures inspired from honeycomb, plant stems and trabecular bone in terms of natural frequency and impact resistance were discovered using machine learning. The discovered optimal cellular unit cells exhibited 30–100% higher natural frequency and 300% higher energy absorption than those of the biomimetic counterparts.
Photo: Mr. Adithya Challapalli, a LAMDA Ph.D. student in the Louisiana State University Department of Mechanical & Industrial Engineering, is using a digital light processing (DLP) printer to print the machine learning optimized lattice core using a TSMP ink.
Impact
Inverse machine learning techniques have been used to discover new molecules, polymers, and drugs. This helped to speed up the process of discovering new materials with superior properties. In our previous work, we developed the inverse design framework to design optimal lattice truss unit cells with superior compression strengths. Here, we used the GAN and regression analysis to design thin-walled cellular unit cells with superior strength and energy absorption capacities based on their natural frequency. The newly proposed cellular unit cells can be used to design lightweight sandwich structures with high energy absorption and damping properties. The inverse design framework can be applied to any other structural designs targeting specific features such as strength, bucking load, acoustic insulation, and many other mechanical, thermal, and electrical features required for a particular application.
Background
Sandwich structures have been widely used in lightweight engineering structures, such as aircraft fuselage, ship hull, car body, wind turbine blade, pressure vessel, pipeline, and bridge deck. The mechanical and functional performance of sandwich structures depends on the core structure. While many core materials have been used such as foam and basal wood, further advancement depends on design and manufacturing of new core structures. Using 3D printing and triple shape memory polymer (TSMP) ink, these lattice cores are manufactured, which is a great challenge using classical reduction manufacturing approach. Therefore, the combination of inverse design using machine learning and 3D printing using TSMP ink opens many opportunities for developing extremely lightweight, robust, and smart sandwich structures of engineering applications.
LAMDA+: Leveraging research projects by engaging on-campus intellectual properties
Saber Nemati and Shengmin Guo, Louisiana State University
When working on an interdisciplinary team, it is very challenging to find a common scientific language between all collaborators. Louisiana State University (LSU) Ph.D. student, Mr. Saber Nemati, analyzed each parties’ needs and developed ideas for sustainable collaborations. The team selected and implemented a sustainable collaboration plan of including LAMDA’s research topics into routine courses that students take in the College of Engineering at Louisiana State University.
Impact
Mr. Nemati’s idea was developed by the LAMDA research team by identifying the intellectual properties at LSU, finding potential candidates and projects, and connecting the interested parties together. On a higher level, this enables the students to become better familiar with the researchers, state-of-the-art research and encourage them to build upon them in their courses. Ultimately, the students may choose advanced manufacturing for their career.
Background
All of the parties involved in LAMDA project have specific needs and motivation. Identifying these needs along with the strength and weakness of each party can help us discover sustainable collaborations. If two parties have at least one common need (and no conflicting need) during a period of time, there is a potential for establishing a sustainable collaboration. For example, faculty members and graduate students both need to have publications. Graduate students bring talent, knowledge, and persistence and faculty members bring research grant and supervision. This can lead to a 2-year or 4-year sustainable collaboration.
Based on the proposed plan, the faculty members can define projects related to the current research project as part of the coursework that the students are required to complete. The research assistant for that particular project can break tasks into smaller components for students unfamiliar with the details of the subject matter so they can successfully complete the project in one semester.
Curing Behavior Simulator for Robotic 3D Printing of UV-Curable Thermoset Polymers
Luke Weger, Luis Velazquez, Corina Barbalata, Debaroty Roy, Armaghan Naderi and Genevieve Palardy, Louisiana State University
A software application to predict and simulate curing behavior of 3D printed filament made from ultraviolet (UV)-curable thermoset polymers is developed. A common cure kinetics model for photopolymers was implemented, as well as experimental characterization data under various UV light intensity levels. Using this custom-designed code, a parametric analysis for various robotic parameters is performed to identify a range of acceptable parameters leading to the design of rigid specimens.
Photo: Mr. Luis Velazquez, a Masters student in the Louisiana State University Department of Mechanical & Industrial Engineering, next to the robotic 3D printing setup for which the simulator was developed, with several printed samples. Mr. Luis Velazquez compares sample quality and dimensional accuracy based on parameters selected with the simulator tool as part of his research for LAMDA.
Impact
UV-assisted printing methods using photopolymers show potential for free-standing printing as material is solidified immediately after deposition. Combining these methods to robotic manipulators demonstrates new prospects for manufacturing of complex 3D shapes, enabling large-scale printing, extensive mobility, and cost effectiveness. However, robotic printing parameters to cure the filaments after extrusion and insure dimensional accuracy (UV light intensity, spotlight diameter, printing speed, nozzle diameter) are typically selected based on extensive trial-and-error approaches. Therefore, this work addresses this limitation to accelerate research on UV-assisted robotic 3D printing. A numerical MATLAB simulator with a user-interface is developed, based on a common cure kinetics model and experimental data for photopolymers, to easily compare the effect of printing parameters on solidification behavior across the extruded filament’s length and thickness. This technique can be adapted to other UV-curable thermoset shape memory polymers (TSMPs) or fiber-reinforced polymers, as well as to other cure kinetics models. It can be combined with 3D printing code to accurately represent printed geometries. As a future optimization tool, it may be integrated with machine learning approaches accounting for material properties (i.e., viscosity) to provide a complete predictive framework including dimensional stability and mechanical performance.
Background
Polymers and their composites (fiber-reinforced polymers) are lightweight materials widely employed in various industries, such as aerospace, automotive, maritime, wind energy, oil & gas, and construction. The mechanical and functional performance of 3D printed thermoset polymers and composites depend on their solidification behavior (also called curing behavior) and micro-structural quality. The proposed simulation framework for UV-curable thermoset printing uses a common engineering software (MATLAB) for which a graphical user-interface was developed. Robotic-assisted printing of high-quality, free-standing structures (solidifying filaments through UV curing as there are extruded) would reduce manufacturing time and costs, and allow geometrical complexity through articulated industrial manipulators.
A soft syntactic foam actuator with high recovery stress, actuation strain, and energy output
Siavash Sarrafan, Xiaming Feng and Guoqiang Li, Louisiana State University
Novel syntactic foams were prepared by incorporating three types of glass microbubbles in a crosslinked cis poly(1,4-butadiene) (cPBD) matrix. Compared to the pure cPBD, which was actuated under 0.32 MPa tensile stress, the foams were actuated under a much larger external tensile stress of up to 1.6 MPa, signifying their superior actuation capability. The energy output is also much higher for the syntactic foam, up to 0.83 MJ/m3, compared to 0.28 MJ/m3 for the pure cPBD. Furthermore, it has a working temperature below zero Celsius and may be actuated at temperatures lower than most other available shape memory polymer foams.
Photo: Mr. Siavash Sarrafan, a Ph.D. student in the Department of Mechanical & Industrial Engineering at Louisiana State University, is preparing syntactic foam by mixing hollow glass bubbles with PBD solution and crosslinker as part of his research for LAMDA.
Impact
Although pure cPBD has exhibited excellent 2W-SME even at frozen temperatures, the main limitation persists in its small actuation stress. If its actuation stress can be further increased and its density can be reduced, it will find many applications in lightweight structures and devices, particularly in cold environments. In this study, a novel syntactic foam with hollow glass microspheres (HGMs) dispersed in cPBD matrix was prepared and tested. HGMs are chemically inert, inexpensive, and have a low coefficient of thermal expansion. With a low density, decent mechanical properties, and great actuation capability, the developed syntactic foams can be adopted for applications such as artificial muscles, biomedical devices, soft robots, sealants, and aerospace structures, which can be potentially manufactured by 3D printing.
Background
Shape memory polymers (SMPs) are a class of polymers that can be programmed to keep a temporary shape and be triggered by mechanisms such as heat or light to switch to the original shape. While one-way shape memory polymers can memorize only one temporary shape, two-way shape memory polymers can memorize two different shapes and can repeatedly shift between the two states. Since the introduction of two-way (2W) SMPs in 2001, many polymers have been developed with the two-way shape memory effect (2W-SME). However, very few of them function in low temperatures. By 2018, there had been only one polymer that could be used in temperatures below 0 °C, and the lowest operating temperature was − 20 °C. However, its actuation strain was limited to only 30% under tension. In 2018, our group reported a 2W-SMP based on crosslinked cis polybutadiene (cPBD) that exhibited an impressive 2W-SME under a maximum tensile load of 0.32 MPa. The cPBD could actuate with an expansion upon cooling (EUC) and contraction upon heating (CUT) over 100%, when temperature cycles between − 40 and 60 °C. More remarkably, this 2W-SMP could function at temperatures much lower than most other 2W-SMPs developed to date. Although the already developed 2W-SMPs exhibit excellent actuation strain, achieving a higher actuation stress could be a substantial improvement as it makes the material even more attractive for applications such as sealants, artificial muscles, and self-healing.
A thermoset shape memory polymer based syntactic foam with flame retardancy and 3D printability
Rubaiyet Abedin and Guoqiang Li, Southern University
A thermoset shape memory polymer-based syntactic foam has been developed incorporating a high-temperature shape memory polymer (HTSMP) as the matrix and hollow glass microspheres (HGM) as the filler. The excellent fire retardancy and 3D printability, together with the high mechanical strength, good shape memory effect, record-high recovery stress, and intrinsic lightweight, make these foams a potential candidate for lightweight structures and devices in several industrial sectors.
Photo: Rubaiyet Abedin, a Postdoctoral Research Associate in the Department of Mechanical Engineering at Southern University, is using a homemade extruder printer to print different specimens using syntactic foam as part of her research for LAMDA.
Impact
In the past three decades, studies have been conducted to investigate the structure-property correlations for syntactic foams and mechanisms for tailoring different properties such as mechanical, electrical, and thermal properties. Combining shape memory polymer as the foam matrix can open up possible load-bearing structural applications, deployable structures, etc. Here, using HTSMP introduces high Tg, flame-retardancy, high recovery stress in the syntactic foam inherently. The foam shows excellent flame retardant performance. Although it may not extinguish the fire completely, it can buy valuable time for firefighters to rescue lives and properties before the structural collapse. We also demonstrated the 3D printability of the foam by an extrusion type of printer with several different structures, including a free-standing structure.3D printability along with the shape memory effect, record-high recovery stress, and flame retardancy can be beneficial in different types of applications in different sectors such as construction, oil & gas, automotive, deployable structures, electronics, marine, and aerospace, etc.
Background
Syntactic foams are polymer composite materials filled with hollow spherical particles such as glass microballoons or microspheres, metallic microspheres, polymeric microspheres, and ceramic microspheres. Compared to open cell foams, closed cell foams or syntactic foams possess high stiffness, low moisture absorption, excellent compressive and hydrostatic strength, and dimensional ability. Syntactic foam has gained popularity for its enhanced mechanical performance and insulating capability and is widely used in different sectors such as marine, automotive, sports, aerospace, deep-sea buoyancy materials, coating, electromagnetic shielding, etc. In this study, we combined an HTSMP matrix along with the hollow glass microspheres. The presence of the hollow space of the microspheres lowers the density of the foam whereas the rigid wall material enhances the stiffness, resulting in high specific properties of the foam. Due to the high recovery stress of the matrix polymer, the syntactic foam also shows high recovery stress. We 3D printed this highly viscous syntactic foam using an extruder developed in our lab. Honeycomb, sinusoidal shapes, and free-standing helical spring were printed for demonstration. Therefore, the combination of high Tg, flame-retardancy, high recovery stress, and 3D printability makes this syntactic foam an excellent candidate in different applications where lightweight, smart foams are required.
Success with Research Experiences for Undergraduate Students During Pandemic Restrictions
Dr. Kristen Williams, Louisiana State University
In light of the pandemic restrictions, LAMDA researchers had to rethink the traditional Research Experiences for Undergraduates (REU) program, and modified it to limited and virtual experiences. The new Extended/Reverse REU program (E/RREU) successfully provided students with meaningful research opportunities and mentoring that fit the social distancing restrictions. In one specific example, Ethan Devillier, a senior at Louisiana State University, participated in the E/RREU program as an undergraduate intern at MELD Manufacturing during summer 2021. He continued research related to his industry experience with Dr. Shengmin Guo during the academic year. His senior capstone design project is sponsored by MELD Manufacturing using a MELD Machine acquired by LAMDA. Ethan is graduating in May 2022 and plans to immediately enter the advanced manufacturing workforce, with intentions to later pursue a graduate degree in materials science.
Photo: Louisiana State University engineering undergraduate student, Ethan Devillier, works on machinery during his internship in Christiansburg, Virginia at MELD Manufacturing Corporation, a subsidiary of Aeroprobe Corporation.
Impact
This experience showcases the benefits of the LAMDA E/RREU program on student education and development to enhance the U.S. manufacturing industry. The E/RREU program develops students into skilled and knowledgeable members of the manufacturing workforce and strengthens partnerships between academia and industry.
Background
Undergraduate students in STEM are the future of the advanced manufacturing industry, and their training is a critical component ensuring Louisiana remains economically competitive in the industry. Industry and academic relationships are key to research advancement in additive manufacturing. The E/RREU program and relationship with industry partners has had direct impact on undergraduate education and outreach and positively contributed to the advanced manufacturing pipeline.
Ethan Devillier’s summer internship gained him invaluable design experience working at an innovative manufacturing company. His knowledge and experience is compounding further through his senior design project and on-campus research experiences, and in addition, MELD Manufacturing has extended an invitation to Ethan to return next summer.
Photo: The collaborative team in front of the MELD L3 additive manufacturing machine (left to right): Dr. Congyuan Zeng, Dr. Shengmin Guo, Mr. Hamed Ghadimi, Mr. Ethan Devillier, and Mr. Brian Baker from Louisiana State University, and Dr. Chase Cox with MELD Manufacturing Corporation.
An Atomistic-informed Continuum Model for Materials Microstructural Design
Ibrahim Altarabsheh, Xiang (Shawn) Chen, Louisiana Tech University
Louisiana Tech University researchers have developed and verified a new computer model that predicts the effect of atomic-details of microstructures on materials mechanical response at a much higher length scale range than previously available. The atomistic-informed Crystal Plasticity Finite Element model was demonstrated on a Cu/Ni bi-material with its interface properties measured from Molecular Dynamics simulations.
Photo: Louisiana Tech University Ph.D. student Ibrahim Altarabsheh conducting materials microstructure design research as part of the LAMDA research team.
Impact
The ability to incorporate the atomic-accuracy into the continuum-level model enhances the predictive capability of complex materials modeling at the mesoscale. It provides a cost-effective approach for materials microstructures design, such as for precipitation strengthening in complex concentrated alloys.
Background
Materials microstructures are often buried in bulk and difficult to access with ordinary experiments. Meanwhile, most of them extend to “mesoscale” (submicron to microns), which is beyond the size limit of typical atomistic simulations while most of the larger-scale modeling alone are no longer accurate or valid. The combination of these two scales in this work meet the challenge in the field for a cost-effective study and design of microstructures for improved material properties.
The National Science Foundation’s support enabled Louisiana Tech University (LATech) Ph.D. student, Ibrahim Altarabsheh, and undergraduate student, Peter Ford, to collaborate with the LAMDA team and to contribute to better understanding of the real-world needs in materials design while receiving training and pursuing their degrees. Ibrahim Altarabsheh submitted an abstract to the U.S. National Congress on Theoretical and Applied Mechanics and will present his work in June 2022. Peter Ford has presented his work at LATech, and will represent LATech at the University of Louisiana System Academic Summit in April 2022.
Room-Temperature Self-Healable and Mechanically Robust Thermoset Polymer for Healing Delamination and Recycling Carbon Fiber
Xiaming Feng, Louisiana State University and Guoqiang Li, Southern University
Here we report a dual-crosslinked network through slightly coupling the low-molecular-weight branched polyethylenimine with an ester-containing epoxy monomer in a non-stoichiometric proportion. The highly mobile and dense noncovalent hydrogen bonds at the chain branches and ends can not only complement the mechanical robustness (tensile strength of 61.6 MPa, elastic modulus of 1.6 GPa, and toughness of 19.2 MJ/m3), but also endow the glassy thermoset polymer (Tg > 40 oC) with intrinsic self-healing ability (healing efficiency > 84 %) at 20 oC. Moreover, the resultant covalent adaptive network makes the thermoset polymer stable to high temperatures and solvents, and yet readily dissolved in ethylene glycol through an internal catalyzed transesterification. The application to room temperature delamination healing and carbon fiber recycling was demonstrated as a proof-of-concept.
Impact
The dual crosslinking strategy proposed here is a fantastic drop-in technology that can be easily used to develop a broad range of high-performance room-temperature self-healable and mechanically robust thermoset polymer. We believe these self-healable thermoset polymers can serve as polymer matrix for fabricating high-performance carbon fiber reinforced composite laminates with practicable self-healing ability in load-carry fields, such as construction and transportation. Moreover, the feasible recyclability enables the recycling and reuse of expensive carbon fibers. We believe that this work could expand the scope of self-healing property and mechanism and create new directions in developing multifunctional thermoset polymers.
Background
The advocacy of carbon neutrality and circular economy encourages people to pursue self-healing and recycling of glassy thermoset polymers in a more realistic and energy-saving manner, the best being intrinsic healing under room temperature. However, the high mechanical robustness and healing ability are mutually exclusive because of their completely opposite requirements for mobility of the polymer networks. So far, to our knowledge, only very few papers reported the intrinsic room-temperature self-healing of glassy polymers. The resulted polymers are rigid yet repairable at room temperature. However, all these polymers still belong to the category of thermoplastics, which are unstable to thermal and solvents. Moreover, the noncovalent crosslinking of networks always means low mechanical strength. Therefore, easily implementing self-healing glassy thermoset polymers for practical applications at room temperature is a grand challenge.
Photos: (A) Photographs illustrating the room-temperature self-healing property: 1) two separate pieces after fracturing, 2) the healed integral after 5 min at 20 oC, 3) the state bearing a weight of 200 g. (B) Digital photos (top row) and SEM images (bottom row) of the new polymer based composite laminate before (left) and after (right) room-temperature self-healing for 64 h: inset image showing the impact damage zone on the back surface.
From drug molecules to thermoset shape memory polymers: A machine learning approach
Cheng Yan, Xiaming Feng, and Guoqiang Li, Louisiana State University
Researchers from Louisiana State University have developed enhanced machine learning, which is able to borrow knowledge from drug molecules to discover the desired new UV curable thermoset shape memory polymers (TSMPs). Firstly, leveraging transfer learning, we trained a variational autoencoder (VAE) model to successively learn knowledge from a big drug database and a small TSMP monomer/crosslinker database. Secondly, we employed 1D convolutional neural network to predict glass transition temperature and rubbery modulus for TSMP, respectively. Thirdly, to validate the models, four different epoxy networks are synthesized. Lastly, we established a large chemical space with 8,019 new TSMPs and screened 5 UV curable TSMPs with desired performance. One of 5 UV curable TSMPs is synthesized and tested to further validate the results.
Photo: Mr. Cheng Yan, a Ph.D. student in the Louisiana State University Department of Mechanical & Industrial Engineering, is designing and encoding the machine learning pipeline for LAMDA.
Impact
This model learns molecule features of TSMP from drug molecules through a machine learning method. Therefore, the model paves the way for machine learning model to extract important features from a small TSMP database and exhibits some superior performance over previous machine learning method in this field.
(a) A crucial limitation for present machine learning design is the database for TSMPs are relatively limited. On the other hand, the databases for drug molecules are plentiful, which are hundred folds greater than TSMP database. With this in mind, we developed a machine learning method to open a channel between two different groups of databases.
(b) One of the barriers for TSMP design with high recovery stress is that they often possess very high glass transition temperature. Therefore, the UV-curable TSMP must be heated up significantly to trigger the SME, but the high trigger temperature may be close to the decomposition temperature of the polymer. Our strategy is shown to solve this problem.
(c) Our model can partially overcome the difficulty of lacking molar ratio information and quickly discovered 5 new UV curable TSMPS with mutually contradictory performances.
(d) We developed a reliable machine learning strategy to extract the really import features from polymer structure.
Background
SMPs are a new branch of smart materials, which can stay in a temporal shape and return to its permanent shape on exposure to multiple external stimuli, e.g., heat or light. TSMPs have found many applications such as crack self-healing in lightweight structures, vitrimer, and deployable aerospace structures, etc. In this study, we would aim to design the TSMPs with high rubber modulus but moderate glass transition temperature, which is limited by two barriers. Firstly, there are only thousands datapoints can be found for TSMPs and thus limits the performance of the model. Secondly, it is dilemma to design the TSMPs with high rubber modulus but moderate glass transition temperature. To solve these, a machine learning method called transfer learning is utilized to solve the problems. With this approach, we are able to leverage the mass data from the existing drug databases and thus significantly improve our model design accuracy.
Illustration: Pipeline for new TSMPs discovery: first, collecting monomer set in the two datasets (c1), and then automatically generating random combinations of monomers and crosslinkers, which produce new TSMPs (c2). Next, fingerprinting is performed for these new TSMPs (c3) and input into the glass transition temperature model (c4) to predict the corresponding glass transition temperature (c5). Then, programming temperature, recovery temperature (c6), can be approximated from glass transition temperature, i.e., Ttr = Tg +20 °C, presumptive uniform strain (c7), and fingerprints of the newly formed TSMP structures (c3) are input into recovery stress model (c8) to predict the corresponding recovery stress σs (c9), which are validated by experiments (c10). Finally, two-step screening processes were conducted. First, if the predicted recovery stress is greater than the maximum recovery stress in the training data, then the corresponding TSMPs are recorded (c11); second, by employing the prior knowledge, promising TSMPs with higher recovery stress than that in the training dataset can be further screened (c12).
Real-time sensors developed to improve quality of 3D printed parts
Arden Moore, Louisiana Tech University
A multi-sensor approach has been developed in which multiple infrared thermal sensors are integrated within a 3D printer in order to detect the occurrence, type, and severity of defects and allow the printer to take corrective action in real-time instead of having to rely entirely on post-print inspection. Having this type of monitoring during a print facilitates higher quality parts, enhanced reliability, and reduced waste of materials and energy.
Photo: Rifat-E-Nur Hossain, a Ph. D. student at Louisiana Tech University and LAMDA researcher, prepares a thermal camera for image capture during operation of a 3D printer.
Impact
Defects within printed parts can lead to reduced strength and early failure, which can be costly and dangerous to end users of additively manufactured components. Even when defects are found directly after printing, the number or severity of defects can require reprinting of the entire part which increases material usage, energy consumption, and lead time. Besides naturally occurring defects, malicious entities may seek to induce defects as a means of sabotaging printed parts via hacking of the printing apparatus. Thus, it is advantageous to implement in-situ condition monitoring whenever possible in order to take corrective measures during the print or abort the print entirely in the case of a critical defect condition.
Background
Most conventional additive manufacturing techniques have limited detection of print process quality during the actual printing process. As a result, defects or abnormalities in the printing process may go unnoticed entirely or are only detected during the post-print inspection. Parts that contain defects that are too severe or too numerous must be scrapped, resulting in the waste of time, material, and energy. The technology and processes being developed in this work seek to change the current paradigm and make in-situ process monitoring accessible for even small printers and across different types of additive manufacturing. Data collection in real-time lets the operator or computer know where a defect has occurred as well as its likely type and size. With additional learning, corrective printing measures may be implemented or the print stopped entirely if the part cannot be saved. In the longer term, in situ process monitoring with real-time defect detection will create new possibilities to establish a self-adaptive system with sophisticated machine learning-enabled prediction features, which will transform additive manufacturing into a more dependable and commercially viable technique.
Synergistic Research, Education, and Outreach through Undergraduate Projects in Additive Manufacturing
LAMDA researchers at the University of Louisiana at Lafayette are utilizing undergraduate students in research and outreach efforts and the students have proven to be highly capable and motivated by the project. 3D printing projects related to our LAMDA research are realizing synergistic benefits in student training, research, professional training, and K-12 outreach in STEM.
Photo: Sample actuator designed and 3D printed by undergraduate students for demonstrating shape memory polymers for K-12 outreach.
Impact
First, the undergraduate students are getting trained with 3D printing, which is a skill that is in high demand by employers, especially since it involves building on other fundamental skills such as Computer-Aided Design (CAD) and materials selection. Second, these students are writing up their work as a research poster for our internal college research competition, giving them practice and experience in research dissemination. Third, the research poster and the samples will be utilized in our College of Engineering’s “Engineering and Technology Day” where our College opens its doors to local high school students to educate and motivate these future engineers to pursue STEM degrees.
Background
Much of the significance and transformative nature of this project relates to the multiple synergistic outcomes from the student training in additive manufacturing. After training, the undergraduate students have fundamental skills for creating 3D printed parts, and their knowledge is then leveraged to promote outreach to local high school students. The students created a poster on what they have learned and will use what they have learned along with the tangible prototypes they have developed to conduct STEM outreach to high school students when they tour our college during our annual Engineering and Technology Day. While instructors and researchers often conduct education, STEM outreach, and research as separate tasks, we have been able to efficiently accomplish all three through this project with a select group of highly motivated students. In the near future, these students will make online videos of these shape memory composites for further outreach to the online community and to supplement outreach efforts by the college.
Cutting-edge technologies for interdisciplinary materials research
by Amber King, Louisiana EPSCoR
Bringing together different disciplines of science is at the core of the advanced manufacturing and materials research effort being conducted by the Louisiana Materials Design Alliance (LAMDA), consisting of researchers from five universities: Louisiana State University (LSU), Louisiana Tech University, Southern University, Tulane University, and University of Louisiana at Lafayette. The alliance is funded by a $20 million cooperative agreement with the National Science Foundation and support from the Louisiana Board of Regents.
This is an exciting era in advanced manufacturing research, bringing together Louisiana’s lead researchers from several disciplines and partnering with industry and national labs. An important part of this research team is graduate and undergraduate students. Louisiana’s bright minds perform critical research under the mentorship of LAMDA’s primary investigators. One of LAMDA’s key student researchers is Mr. Saber Nemati, a PhD student at LSU.
Photo: LAMDA PhD student Saber Nemati working with Avizo for 3D reconstruction of tomography data at LSU.
Nemati researches how to detect materials defects inside of advanced manufactured parts. “Currently, I am working on the applications of machine learning in materials engineering. Particularly, we are trying to apply novel intelligent methods for real-time monitoring of additive manufacturing parts using synchrotron/neutron beamline tomography for material characterization and microstructure analysis. In other words, we want to create an ‘artificial materials specialist’ who is as smart as an experienced engineer and as fast as a computer, and of course does not face the common safety issues that humans do,” said Nemati.
“This is not only a hot field of study, but also an exceptional opportunity to deal with today’s technological trends in research and development,” added Nemati.
This research is truly interdisciplinary, combining theoretical, experimental and numerical aspects of materials science, engineering, computer science, and physics fundamentals. Nemati belongs to a team who designs different polymer and alloy materials, algorithms, testbeds and specimens and then tests their strength with supercomputers. After the virtual testing, the experimental results are physically tested in the lab to verify the results.
Nemati works with Dr. Shengmin Guo, an expert in Additive Manufacturing, Dr. Xin Li, who is known for his expertise in Image Processing, and Dr. Les Butler whose research is focused on Tomography. “It is a great honor and a big opportunity for me to work under the supervision of these acknowledged experts,” said Nemati.
Photo: Saber Nemati performing high frequency ultrasonic fatigue testing of small key-shaped specimens for evaluating fatigue life of additive manufactured parts.
“Is there any way to do this better?”
This was the recurring question that Nemati would ask himself, as a young student who loved puzzle magazines and, later as an engineer, in ordinary situations, such as riding a public bus and thinking about improving the paper ticket process. This mindset led him to study mathematical sciences in high school and mechanical engineering in college in Iran where he grew up.
After acquiring the basic knowledge earning his undergraduate degree and a masters degrees in Mechanical Engineering, he broadened his experience by working in industry and facing the challenges of real-world problems. He later felt the need for a broader perspective, and earned his MBA with a focus on Strategic Management, which helps him have a bigger picture of the whole process, from identifying customer needs to production process to sales and marketing.
Ultimately, he wants to be an entrepreneur who can develop a sustainable relationship between science and industry. He has persistently paved the way to achieving this goal during his education and career.
He is currently working toward his goal of completing his PhD in Engineering Science at LSU. He decided on attending LSU when he visited Dr. Guo’s webpage, and saw that under the research goal it was written, “Provide challenges and opportunities for future engineers and high-tech leaders.”
“I believe with his attitude, no other mentor can contribute more to my decision than him,” said Nemati.
“The most challenging and exciting skill that I’m learning in this project, is having the ability to communicate with various scientists and experts from different backgrounds.
Normally, computer scientists and materials engineers do not have so much in common. But with LAMDA and its interdisciplinary nature, it is absolutely crucial to learn how to maintain a scientific communication among different groups,” concluded Nemati.
Accelerating deep learning discovery of new thermoset shape memory polymers
Cheng Yan, Xiaming Feng, Louisiana State University; Collin Wick, Andrew Peters, Louisiana Tech University; Guoqiang Li, Southern University
Researchers with the Louisiana Materials Design Alliance (LAMDA) established a machine learning framework to predict the recovery stress of thermoset shape memory polymers (TSMPs) and to discover new TSMPs with superior recovery stress. The team leveraged a new linear notation computer language for the digital representation of polymers, called BigSMILES, to fingerprint complex TSMP structures and establish structure-property correlations using a small training dataset. This information helped to identify two new TSMPs predicted to have high recovery stress, which was synthesized in the lab to validate the model predictions. Finally, they explored a chemical space with 4,459 possible TSMPs and screened 14 mostly unknown TSMPs with higher recovery stress than any TSMPs in the training dataset. One of 14 TSMPs was modeled by molecular dynamics simulation and found to have calculated recovery stress in agreement with predicted values.
Mr. Cheng Yan, a Ph.D. student in the Department of Mechanical & Industrial Engineering at Louisiana State University, is designing and encoding the machine learning pipeline.
To our knowledge, this study is the first to discover TSMPs with high recovery stress by leveraging machine learning. Using a small dataset of about 100 molecules, this method has the potential to greatly increase the ability to explore chemical space and bring remarkable advancements over previous materials discovery methods.
a) A bottleneck for current TSMPs persists in their low recovery stress in their rubbery state, limiting their applications as actuators or as crack closing devices in self-healing applications.
b) Due to the time it takes to synthesize new TSMPs, the traditional trial-and-error method for materials discovery needs a long period of time, deep domain knowledge and skills, and some luck. This new method overcame these limitations and quickly discovered 14 new TSMPs.
c) The machine learning approach is at least hundreds of times faster than the traditional molecular or atomistic computational approaches, such as molecular dynamics simulation and density functional theory based electronic calculation.
Traditionally, predictions of thermomechanical behaviors of TSMPs, such as recovery stress, rely on multi-parameter constitutive models, and most of the parameters need to be determined by curve-fitting. This machine learning model, on the other hand, only depends on the basic chemical structures and so can be applied nearly universally.
TSMPs are a new class of smart polymers, which, after deformation, can maintain their deformation nearly permanently until a stimulus, such as heat or an electric field, is applied. This stimulus causes the deformed TSMP to restore its original shape. TSMPs have found many applications, such as crack self-healing in lightweight structures, stents in medical applications, and artificial muscles in soft robots. However, a persisting critical limitation of existing TSMPs is their very low recovery stress in their rubbery state, usually less than a few Newtons per square millimeter. This recovery stress is too low for some critical applications, such as the crack closing. It is also too low to compete with shape memory alloys, which may have tens to hundreds of Newtons per square millimeters in recovery stress. Unfortunately, the chemical space constituting TSMPs is very large, and using trial and error approaches is not sufficient to identify new TSMPs with higher recovery stress. Therefore, machine learning is a natural choice. As compared to conventional polymers—which have already had a large database in Materials Genome Initiative—only a limited number of TSMPs are available for training and fingerprinting. In this study, we successfully overcame this limitation and discovered 14 new TSMPs. This machine learning approach can be expanded to discover other types of materials.
Pipeline for new TSMPs discovery: first, collecting monomer set in the two datasets (c1), and then automatically generating random combinations of monomers and crosslinkers, which produce new TSMPs (c2). Next, fingerprinting is performed for these new TSMPs (c3) and input into the glass transition temperature model (c4) to predict the corresponding glass transition temperature (c5). Then, programming temperature Ttr, recovery temperature (c6) (approximated from glass transition temperature, i.e., Ttr = Tg +20 °C), presumptive uniform strain (c7), and fingerprints of the newly formed TSMP structures (c3) are input into recovery stress model (c8) to predict the corresponding recovery stress σs (c9), which is validated by experiments (c10). Finally, two-step screening processes were conducted. First, if the predicted recovery stress is greater than the maximum recovery stress in the training data, then the corresponding TSMPs are recorded (c11); second, by employing the prior knowledge, promising TSMPs with higher recovery stress than that in the training dataset are be further screened (c12).
Phosphate esters dynamic chemistry enables flame retardant vitrimers
Xiaming Feng, Louisiana State University and Guoquiang Li, Southern University
Researchers with the Louisiana Materials Design Alliance (LAMDA) report the first phosphate esters based thermoset polymers that are mechanically strong, completely malleable and recyclable, and significantly safe under fire. These promising properties rely on the unique behaviors of β-hydroxy phosphate esters at low, medium, and high temperatures, respectively. At room temperature, the abundant hydrogen bonds in the network contribute to outstanding toughness (5.44 MJ/m3). Around 100 oC, the catalyst-free rapid exchange reaction between phosphate esters and neighboring β-hydroxyls endows the polymer with almost 100% recycling efficiency. Above 250 oC, a cellular layer of charred phosphoric acid generated from β-hydroxy phosphate esters could separate/insulate the heat effectively, providing fire protection. In addition, by combining phosphate diesters and acrylates, a new polymer integrated with ultraviolet (UV) curability, recyclability, and flame retardancy are also developed. This highly crosslinked network exhibited attractive recyclability even at the temperature lower than glass transition temperature. The fast exchange reactions via catalyst-free mixed transesterification between phosphate diesters and carboxylate esters of acylate structures are validated.
(Top row) The broken thermoset vitrimer pieces after service can be reshaped into a new transparent sheet by a simple hot pressing for reuse. (Bottom row) The vitrimer sheet under the cotton ball generates an expanded char layer upon external fire to protect the cotton ball from temperature rising and burning for a couple of minutes.
The phosphate dynamic chemistry proposed here is a fantastic drop-in technology that can be easily used to develop a broad range of high-performance vitrimers while possessing intrinsic flame retardancy. Coupled with the high transparency, these self-healing fire-safe vitrimers can serve as multifunctional coatings for metallic structures or components with a high risk of fire and corrosion, such as in construction fields and electronics. The UV curability of the combination of phosphate diesters and acrylates enables the printing of customized and complicated structures in these advanced fields, such as robotics and aerospace, using digital light processing (DLP) technology. We believe that this work could expand the scope of dynamic covalent chemistry and create new directions in developing multifunctional thermoset polymers.
Fire hazards are a well-known limitation for polymers. Thermoset polymers, while they have high mechanical strength and thermal stability, are usually not recyclable, which causes a significant waste disposal issue. Therefore, sustainability and safety have been one of the key issues in polymer science and engineering due to the shortage of natural resources, the crisis in waste disposal, and fire hazards caused by flammable polymers. Recyclable thermosets or vitrimers and fire-retardant polymers have been developed separately for years to address these challenges partially. To our knowledge, no other vitrimer has demonstrated fire-retardant capability without adding extra flame-retardant structures. Therefore, integrating robust mechanical performance, recyclability, and flame-retardancy into one polymer using the new facile dynamic covalent chemistry initiated in this study is of significant value to both academia and industry.
Recyclable Composites: The future of lightweight materials
John Konlan, Louisiana State University; Sam Ibekwe, Patrick Mensah, Karen Crosby, Guoquiang Li, Southern University
Researchers with the Louisiana Materials Design Alliance (LAMDA) developed a new self-healable and recyclable fiber reinforced thermoset shape memory vitrimer composite laminate. In this study, they used pre-tensioned shape memory alloy (SMA) reinforcing fibers called “z-pins” to control delamination, which is cracking at the interface between neighboring layers, and used the thermoset shape memory vitrimer for molecular-scale healing of the delamination. The team found significant enhancement in low velocity impact tolerance, and high healing efficiency even under repeated impact damage and healing cycles for the laminated composite.
Mr. John Konlan, a Ph.D. student in the Department of Mechanical & Industrial Engineering at Louisiana State University, is examining the self-healing composite laminate.
In recent years, self-healing and recycling of fiber-reinforced polymer composites have become a popular topic of research. However, most of the studies are either focused on damage healing of pure polymers or limited to damage healing of polymer composites with microscale cracks. In this study, by a novel combination of SMA z-pins and thermoset shape memory vitrimers, we are able to repeatedly heal wide-opened delamination in glass fiber reinforced vitrimer composites. The combination of SMA, shape memory and thermoset vitrimer opens up a new opportunity to develop the next generation of laminated composites for lightweight structural applications.
Delamination induced by low velocity impact: (left) with SMA z-pins and (right) without SMA z-pins.
Continuous fiber-reinforced polymer composite laminates have been widely used in lightweight load-bearing structures. It is well known that laminated composite is vulnerable to impact damage. For example, even a drop of a hammer during a routine inspection of laminated composite structures represents a low-velocity impact event, which may induce delamination, matrix cracking, matrix/fiber interfacial debonding, and fiber fracture. Among them, delamination can reduce the compressive load carrying capacity of the composite by over 50%. On the other hand, recycling of the end-of-service laminated composites poses a solid waste disposal issue. Therefore, how to make a laminate composite that is self-healable, recyclable, and impact tolerant is highly desired. In this study, we used a combination of SMA z-pins and thermoset shape memory vitrimer to prepare SMA z-pinned, continuous glass fiber reinforced vitrimer laminated composite. The results show that SMA-pins have significantly reduced the delamination opening (from > 0.2 mm for those without SMA z-pins to < 0.03 mm for those with SMA z-pins), and the vitrimer matrix can be healed and recycled repeatedly under multiple low-velocity impact cycles.
Inverse machine learning technique optimizes discovery of materials for sandwich structures
Adithya Challapalli, Louisiana State University and Guoqiang Li, Southern University
Researchers with the Louisiana Materials Design Alliance (LAMDA) have developed an inverse machine learning approach using the generative adversarial network (GAN) and regression analysis. Using this technique, novel lightweight lattice and cellular structures with optimized biomimetic elements are discovered, which are used as sandwich core. Thermoset shape memory polymer (TSMP) is then used to 3D print these designs, which adds smartness to the sandwich structures.
Mr. Adithya Challapalli, a Ph.D. student in the Louisiana State University Department of Mechanical & Industrial Engineering, is using a digital light processing (DLP) printer to print the machine learning optimized lattice core using a TSMP ink.
Inverse machine learning techniques have been previously used to discover new small molecules, polymers, and drugs. This helped in speeding up the process of discovering new materials with superior properties. Here, we used GAN and regression analysis to learn the correlation between the microstructures and mechanical properties of biomimetic rods and then further optimized the biomimetic rods into lattice and cellular structures as a sandwich core. 3D printing of the newly discovered lattice core by TSMP makes the new core with robust structural properties and shape memory effect. This technique can be applied to any other structural designs targeting specific features such as strength, bucking load, vibration damping, acoustic insulation, and many other mechanical, thermal, and electrical features required for a particular application. By further generalizing this inverse design technique, it can be incorporated into existing computational and simulation software as a futuristic optimization tool or as an individual application that can predict optimal designs.
Step-by-step optimization by machine learning for discovering lattice core and thin-walled cellular core for lightweight sandwich structures.
Sandwich structures have been widely used in lightweight engineering structures, such as aircraft fuselages, ship hulls, car bodies, wind turbine blades, pressure vessels, pipelines, and bridge decks. The mechanical and functional performance of sandwich structures depends on the core. While many core materials have been used, such as foam and basal wood, further advancement depends on the design and manufacturing of new core structures. Here, we use a combination of GAN and regression analysis and optimize lattice and cellular cores for sandwich structure with biomimetic rods, which is infeasible using the classical design approach. Using 3D printing and TSMP ink, these lattice and cellular cores are manufactured, which is a great challenge using the classical reduction manufacturing approach. Therefore, the combination of inverse design using machine learning and 3D printing using TSMP ink opens up many opportunities for developing extremely lightweight, robust, and smart sandwich structures for engineering applications.
New specimen design improves rapid fatigue testing
Hamed Ghadimi, Louisiana State University
A new small-sized test specimen has been designed by researchers with the Louisiana Materials Design Alliance (LAMDA) to accelerate bending-fatigue testing. Small-sized test pieces are more adaptable, economical, and timesaving in fabrication processes and contain quantifiable imperfections and defects, which makes them highly desirable for rapid qualification of new alloys and new fabrication processes.
Louisiana State University graduate student Hamed Ghadimi working with SHIMADZU USF-2000 ultrasonic fatigue testing system.
This new design of a small-sized test specimen enables fatigue property studies of new 3D printed alloys to be carried out rapidly and with significantly reduced cost. Rapid fatigue testing capability helps scientists to explore new alloys and 3D printing techniques for reliable and durable products.
Bending-fatigue testing setup and designed small-sized test specimen.
Fabrication of test pieces and doing experiments on them is important, particularly for additive manufacturing (AM) studies, like developing new AM alloys, investigating proper AM design, and studying the effects of AM build parameters on the mechanical properties. Fatigue testing is an essential part of such studies, and the high-cycle life regimes are time-consuming and costly, and a large number of test specimens with a suitable design are needed. For AM research, scientists also encounter severe restraints such as limited building-capacity, small chamber size, high costs, and unpredictable microstructure defects. Ultrasonic high-frequency fatigue testing systems overcome the time- and cost-related limitations, and in addition, reducing the size of the test specimens is a way to tackle the above-mentioned obstacles. Small-sized test specimens are also beneficial from the affordability point of view as they make the controlling of the manufacturing processes more feasible, and thus, they facilitate the production of more qualified specimens under a variety of different processing conditions.
Developing artificial intelligence for defect detection
Saber Nemati, Hao Wen, Brian P. Tsai, Louisiana State University
Supervised Machine Learning (ML)-based algorithms are being developed by researchers with the Louisiana Materials Design Alliance (LAMDA) for crack detection in X-ray tomography images. Using this method, a large number of images can be segmented with accuracy after training with just a limited number of manually segmented images.
Louisiana State University graduate students, Saber Nemati, Hao Wen and Brian P. Tsai evaluating different network architectures.
The ability to efficiently detect the cracks and different types of inclusion in tomography images is a key component in evaluating and optimizing additively manufactured (AM) parts in the testing stage. This paves the way for developing faster and more intelligent algorithms with minimal supervision, which consequently leads to a more optimized way of designing stronger, premium alloys.
Sample algorithm segmented image. (a) original image (b) Fe-Inclusions (c) Mg-inclusions.
Creating suitable additively manufactured alloys for different applications is a challenging task, as it depends on many parameters during the designing, manufacturing, and testing processes. One of the most challenging phases of this process is the evaluation of the parts since defects in AM parts are hard to detect and cumbersome in nature. Tomography is a well-known nondestructive method for observing the defects inside 3D metal parts on micro scale. Due to recent advances in machine learning, there are a lot of efficient algorithms that can identify these defects and categorize them into different classes. With the implementation and validation of such algorithms, several days of manual work can be completed in a couple of seconds. Because of LAMDA’s interdisciplinary nature, the collaboration of experts with different scientific backgrounds is inevitable. One of the most valuable yet challenging outcomes of LAMDA is building a synergetic framework that makes use of each groups’ capabilities.
Virtual Training Modules for Additive Manufacturing Bridges Knowledge Gaps During COVID Closures
Mohammad Khondoker, Southern University
A group of 72 attendees, including faculty members, graduate/undergraduate students, participated in the workshop to learn about all seven categories of additive manufacturing technologies and their underlying process physics.
Additive manufacturing development opportunities serve as a crucial early component for the workforce development initiatives aimed to benefit the U.S. manufacturing industry. In addition, faculty members and researchers from other disciplines have also gained the necessary knowledge to advance their research. More than 10 LAMDA-affiliated faculty members earned in-depth knowledge on additive manufacturing which will be useful in performing LAMDA’s research activities (both SD-1 and SD-2), as well as in developing new grant proposals in that field.
Coursework that covers all seven categories of additive manufacturing is not available at universities in Louisiana. Therefore there was a need for a workshop training module to train graduate and undergraduate students in Louisiana. Through this workshop, more than 15 graduate students and 35 undergraduate students received the necessary knowledge to prepare them for the manufacturing industry in Louisiana.
Additive manufacturing (AM) is one of the core components of the fourth industrial revolution, called Industry 4.0. Therefore, it is essential that the U.S. manufacturing industry is supplied with a trained workforce that can utilize the true capabilities of AM technologies. There are seven different types of AM technologies with unique advantages/disadvantages. A manufacturing engineer needs to know the principles of these technologies and their underlying process physics, which will help in improving the performance and lowering the cost of manufactured parts. Being a relatively new technology, AM is not well understood by students because most programs in higher education do not offer AM-related courses. Hence, arranging workshops to educate graduate/undergraduate engineering students on AM technologies plays a vital role in workforce development for the U.S. advanced manufacturing industry. Such initiatives will help the U.S. to remain the global leader in this field.
2020 and earlier
LAMDA is built upon a foundation of decades of research and leverages current and previous awards and infrastructure investments to continue the growth of materials science research and industries in Louisiana. This foundation was boosted by two previous NSF Track-1 RII awards, the Consortium for Innovation in Manufacturing and Materials (CIMM), and Louisiana Alliance for Simulation-Guided Materials Applications (LA-SiGMA).
View a compilation of research highlights from LAMDA, CIMM and LA-SiGMA.